Better data lead to better forecasts
The challenge is knowing what are good data and where to find them
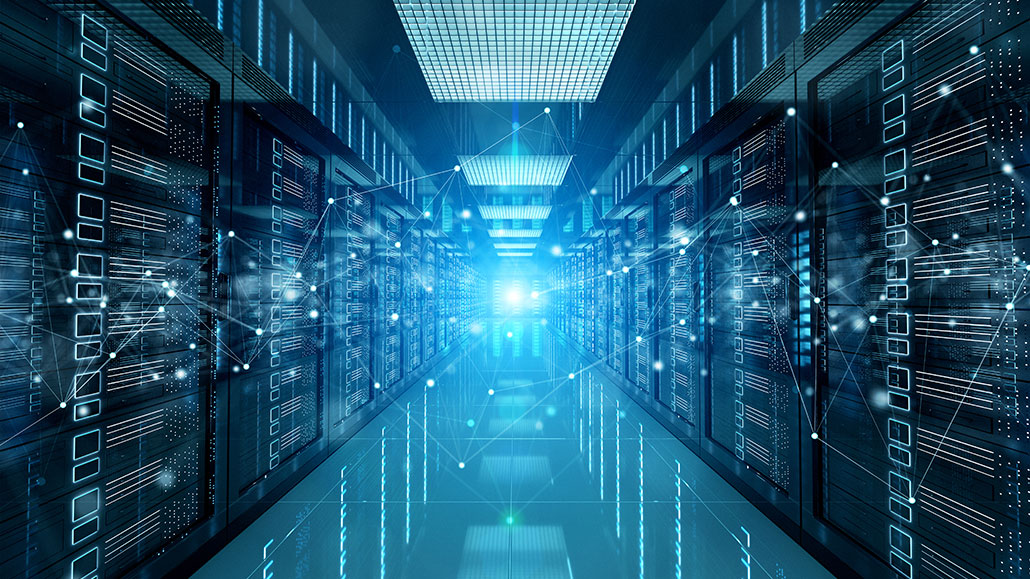
Computer servers (such as those seen in this illustration) store mountains of data. And with the appropriate math, some of those data can be turned into useful information that can help forecast future events.
sdecoret/iStock/Getty Images
You may have heard this phrase that computer scientists use all the time: Garbage in, garbage out. It means that a computer will do all it can to answer a problem, but if you don’t feed it good starting material, don’t expect a quality solution. And the same is true for mathematical models performed on or off a computer. These models need data to work, and if the data aren’t very good, the model’s forecast won’t be either.
And that immediately raises the question: Where do you find good data?
Weather stations across the globe gather mountains of good data for weather-forecasting models. Satellites collect additional data about the atmosphere and the ocean. Instruments on airplanes or carried by balloons provide still more measurements of temperatures, wind speeds and precipitation.
Caches of data can be found throughout the internet. Governments collect some data sets. Others come from organizations, universities, even individuals. Some of these sets include enormous mountains of data.
Data can come from anywhere. Want to know how many people go on cruises each year? Or how often Europeans go to church? Or how many Americans voted in the 1972 presidential election? Those are among the data that can be found.
Emily Kubicek is a data scientist in the Los Angeles, Calif., area. She works for the Walt Disney Company in their Disney Media and Entertainment Distribution business segment. Earlier in her career, she worked for the National Deaf Center in Austin, Texas. There, she gathered data on both hearing and deaf Americans to see how the two groups compared. The data came from the U.S. Census Bureau.
Those Census data are free and available to anyone. They also represent the nation as a whole. Kubicek mined these data for details, such as how much schooling people had. What jobs were most popular in each group? What languages did people use? Her group also looked at whether there were any patterns in how such traits have changed over time.
Many scientists get their data from Kaggle. This online community of scientists shares huge amounts of data. For instance, it’s one place to find huge sets of data from the University of California, Irvine’s Machine Learning Repository.
Sometimes, though, the data researchers want can be hard to find. Natalie Dean is a statistician at the University of Florida in Gainesville. Lately, she’s been working on predictions about the spread of the new coronavirus. Since this virus that causes COVID-19 is so new, there are relatively few data about it. “We’re still learning things about this particular virus,” Dean says. So in contrast to the weather, which has been studied for many decades, “there are more uncertainties [about this virus].”
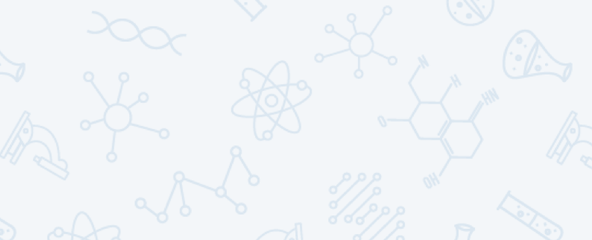
Educators and Parents, Sign Up for The Cheat Sheet
Weekly updates to help you use Science News Explores in the learning environment
Thank you for signing up!
There was a problem signing you up.
Experts needed
How people make decisions can complicate which data will be useful, too. “Sometimes we look at data about where people are going. We use apps like Google Mobility and Foursquare,” Dean says. These apps track people’s movements. Still, they tell you only so much. “You can see that people are going to restaurants less. But you don’t know if when they go they’re wearing masks and staying distant from each other.” And are they meeting up with friends they haven’t seen in a while or going with household members? Those might be important questions if you want to know how this behavior affects the spread of COVID-19.
And no computer model can do all the work for you. First, you have to decide which data are important, notes Michael Lopez. He’s a statistician for the National Football League, in New York City. You can’t just throw in a bunch of random sports statistics into a model, he says, and expect it to tell you if the particular running back you’re scouting for a team will be successful. You need to include just the right data. And humans have to figure that what those will be.
Kubicek at Disney calls this “domain knowledge.” The domain is the field you’re working in — say climate, sports or some infectious disease. To build good models and interpret their results, you have to know a lot about the field. This is why many of the best statisticians aren’t just math whizzes. They are also experts in their fields.
Before becoming a statistician with the NFL, Lopez played and coached football. In college, Dean majored in math and biology but found that she didn’t like working in a lab. When she took a statistics class, she discovered she could combine her two interests. Kubicek always loved science, but was rarely encouraged to study it. While studying to be a speech therapist, she got a job that showed she was really good at science. So she switched course and got a PhD in neuroscience. Then she taught herself to code. Her mix of interests has made her a good forecaster.
With their expertise, they can check that the data they put into a model aren’t garbage. After all, they want what comes out to be the equivalent of a reliable gourmet meal.