Computers can translate languages, but first they have to learn
Google Translate learns just like a baby — except this baby eats its words
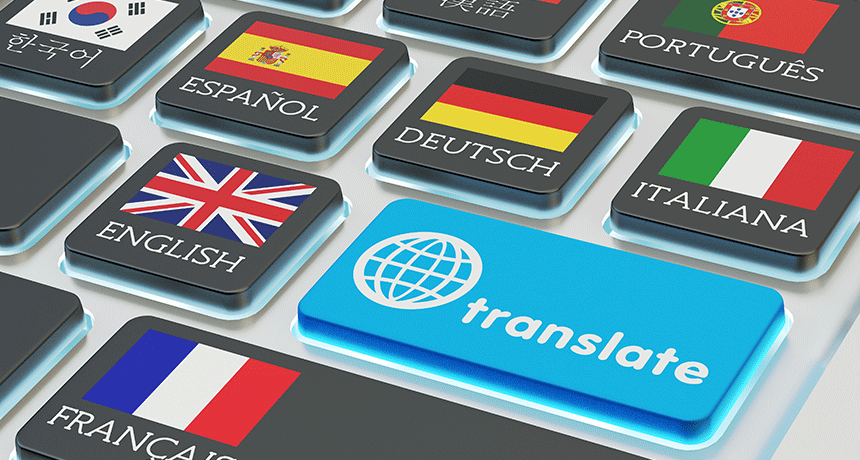
If you want to know what a bit of French, Japanese or another foreign language means in English, it’s easy to get a translation through a website, such as Google Translate.
cybrain/iStockphoto
By Terena Bell
A Canadian family is traveling overseas with children when one of them whispers to her mom that she really, really, REALLY has to use the washroom. In other words, it’s an emergency. But how does this parent ask for the location of the restroom from someone who only speaks Turkish, Portuguese or Mandarin?
With a smartphone, that mom might be able to ask for help from Google Translate. The website lets someone type a word, phrase or more in English. Then it shows the equivalent words in Spanish — or any of more than 100 other languages. When people say “Google Translate,” they sometimes mean this website. Other times they may be using the phrase to inaccurately refer to any machine translation engine, which is what these computerized programs are known as. (Calling all of them Google Translate is like using the brand-name Kleenex to refer to any brand of tissues.)
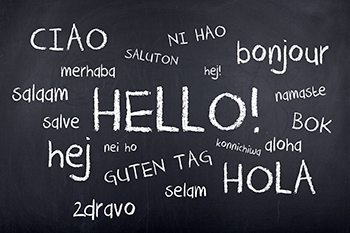
Machine translation — or any type of translation, really — isn’t easy. Languages never match each other word for word. For example, think about the word “honeymoon.” This doesn’t mean that the moon is made of honey. If you want to say “honeymoon” in another language, you first have to think about what it really means: a trip people take after their wedding. That’s why the way you say “honeymoon” in French is “voyage de noces” — which literally means ”wedding trip.”
Meaning is more important than exact words when it comes to translating something into English, too. Pretend you’re French and your big sister is talking about her date. “You’re not going to believe it,” she says in French, but “he gave me a rabbit.” Of course, Frenchmen don’t show up for dates bearing bunnies, usually. The phrase — “Il m’a pose un lapin” — is a French idiom. It means “he stood me up.”
If translation challenges people, imagine how tricky it can be when teaching a computer to do it. And it has been hard. But programmers have been making great strides lately in helping electronic brains interpret “foreign” languages.
Here we look at many of the obstacles they’ve had to hurdle. Fortunately, the payoff for succeeding can be big: Different cultures will not have to give up their own languages — or, necessarily, learn a new one — to be understood throughout the world.
Learning the rules . . . and when to break them
Machine translation systems learn like a baby does. Let’s name ours MT. That’s the abbreviation professional translators actually use.
Just like a baby, MT has a brain. In machine translation, this brain is known as an engine. Such engines are completely empty until fed words. Words such as “honeymoon” sometimes will be eaten in isolation. Others may be ingested in phrases or sentences such as: “My mom and dad went to Gatlinburg on their honeymoon.”
MT’s “brain” processes these words in one of two ways: rule-based or behavior-based. It takes both types to understand language. (That’s true both for computers and for people.)
Think about it. People talk in full sentences around babies all the time. Sure, they may say “apple” over and over again while pointing to one. But they also say, “Wow, MT, you really like your apple.” By seeing how words work within the context of the other words in a sentence, MT’s brain starts growing slowly. This may start with learning the meaning of “apple.” To do this, MT learns a bit each time the word is used. But just as importantly, it learns something by noting when the word is not used.
For instance, you would never say: “My parents went to Gatlinburg on their apple.” But you could say “They went to the Big Apple on their honeymoon!” Since words can mean more than one thing (Big Apple is a nickname for New York City), behavior-based engines help MT understand the use of specific words — here, “apple” — in a range of different situations.
But such behavior-based rules are not enough. That’s why rule-based engines exist. They help MT understand the grammar of a particular language. For example, those rules teach MT what a noun is. They teach how a noun differs from a verb, and that in English, nouns go in front of or after the verb depending on how it relates to the verb. So rule-based engines help MT know not just that “apple” is a noun but also that “I want an apple” works but “I apple a want” does not.
Good grammar helps you sound as smart and educated as you really are. But sometimes you may just want to sound cool. To do that, you might deliberately bend — or even break — a rule.
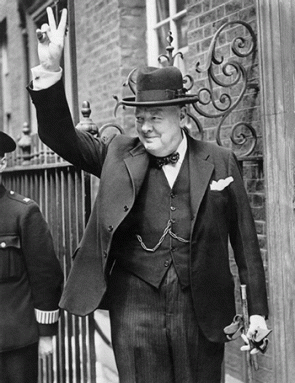
For example, experts in English grammar know that people should not end a sentence with a preposition (such as “of” or “to” or “with”). Yet people do it all the time. Even Winston Churchill did it. He was Prime Minister of the United Kingdom and a renowned public orator. His motivating speeches are credited with helping the Allied forces win World War II.
Once, Churchill took some criticism for ending a sentence with a preposition. His response: “Ending a sentence with a preposition is something up with which I will not put.” Instead of saying “something I won’t put up with” — which is what people normally would say — Churchill instead uttered a silly phrase. He wanted to point out that you can sometimes sound weird when you don’t break the rules.
The problem, however, is that computers can’t break the rules. They don’t know how. Even those that have artificial intelligence can break rules only in ways that they were taught.
People break the rules to make up new sentences and words all the time. For MT to understand and do that, it must learn the rules for when rules can — or should — be broken. This is why a good MT engine really needs both behavior and rule-based learning. The beauty and usefulness of language depends on both.
Words as food
An engine that learns from both rules and behavior is called a hybrid. Computer programmers train such hybrids by using larger chunks of information, not just isolated words or phrases.
“Most of these hybrids learn from data,” explains Kirti Vashee. He is a private consultant, based in Los Angeles, Calif. He helps translation companies build their own engines. MT developers would likely start by feeding an engine huge quantities of previously translated words and phrases. They provide a young MT engine with a digital library of translations from one particular language into another. By absorbing these data, an MT system will become more articulate and educated in the grammar of this language.
In the past, MT programmers trained engines with entire books. Books not only contain sentences that follow grammar rules, but also sentences that don’t. The more of both that programmers feed into an MT engine, the more it will learn. A machine translation company known as Sovee, for instance, included the Bible among the texts it used to train its MT engine.
Machine translation does not perform equally in all languages. Why? The simplest answer is that no engine will have been fed a similarly rich diet of every language.
Mike Dillinger oversees artificial-intelligence efforts at LinkedIn, the social media network based Sunnyvale, Calif. He’s also on the board of the ADAPT Centre in Dublin, Ireland. “We train each MT system to go in only one direction,” he explains. That “language direction,” for example, might be from English into Spanish. “We then have to train a separate one,” he notes, “if we want it to go in a different direction, for example, from Spanish to [English].”
Some languages, like Spanish and French, have rich literary histories. With many books written in these languages, MT has a large and varied diet of words on which to dine. Far more books and other written works also exist that have already been translated between these languages and English.
Compare that to Somali. This language is spoken in the African countries of Somalia, Djibouti, Eritrea, Kenya and Ethiopia. Until 1962, no one had even written this language down! For the next decade, political leaders in Somalia fought over what their alphabet should even look like, notes Mohamed Diriye Abdullahi. (He wrote the 2001 book Culture and Customs of Somalia.)
Compare this to English. The first written text in this language appeared in 602. That’s 1,370 years prior to written Somali. Even today, people who speak Somali don’t always agree on how to spell certain words. So, with fewer texts and with different spellings for words, you can see how an MT engine might get confused!
Yet even when plenty of books are available, they sometimes “aren’t about the right topics,” Dillinger points out. Besides needing an engine for each direction, MT may work best if it has separate engines for different subjects. For example, one English-to-Spanish engine may translate about food while another translates about sports.
“We have to choose example sentences that are the same topic and same style that we want the translations to look like,” Dillinger says. After all, “We don’t teach the computer a language,” he explains. “We show it some example sentences to copy.”
Story continues below video
Don’t feed your engine ‘junk food’
Like a baby, an MT engine has to be exposed to a lot of words to understand just a few very well. Once it does, though, MT’s ability to translate grows in proportion to how much it’s fed — and at an accelerated rate. Why? Because MT gets smarter from every interaction.
Every time someone uses a young MT engine, they feed it words. Humans don’t just eat until they grow up. They eat until they grow old and die. So an MT engine also needs many words to begin growing — then plenty more to help it mature and get even better at its job. Indeed, feeding an “adult” MT engine is quite important. The reason: Over time languages evolve and grow. New slang terms emerge. People give new meanings to old words. And totally novel terms are invented to describe new events, technologies or concepts, such as synestia. MT engines must grow and evolve to account for all of these.
Languages that Google doesn’t translate very well, such as Somali, tend to be spoken in countries where there are fewer texts and where it can be hard for people to get access to the internet. So they don’t offer Google Translate much to eat. An MT engine trying to learn such a language may therefore remain stunted for many years.
After all, Google Translate — or any MT engine — should get smarter and faster the more it eats. But only if it eats real words.
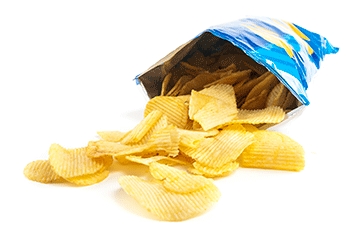
Keep in mind that MT will eat anything it’s fed. This is why you should never type made-up words into such an engine. Every time you do, you’re stuffing MT full of the linguistic version of potato chips instead of fruits and vegetables.
Google has found that people already type more than 140 billion words into Google Translate every day! At this rate, its MT engine is definitely no longer a baby.
But bigger doesn’t always mean stronger.
“Large volumes of bad data will produce crappy MT systems,” Vashee says. In other words, feeding MT weird, odd or fake words makes it fat and unhealthy. So every time you use Google Translate or some other computerized translation program, think of yourself as its parent. Are you feeding this MT healthy words or the equivalent of junk food?
Unfortunately, people are going to keep typing made-up things into Google Translate for fun. Until MT learns how to toss these words out instead of eating them, it may suffer. Junk food slows you down — and risks doing the same thing to MT.
Bringing machine translation to everyone
Everyone deserves to have computers (and other people) understand them. So computer programmers are always looking for ways to make MT smarter and more accessible. The challenge can be to make this happen for the many “tongues” — such as Somali — with fewer written texts or fewer speakers.
Laura Brandon works in Seattle, Wash., where she is director of the Globalization and Localization Association. It’s the international trade association for translation companies. Computer programmers have been building computer-based language translators since the 1960s, she notes. “The big development these days,” she observes, “is neural MT.” Neural here refers to neurons in the brain. And although MT engines lack true neurons, they have been designed to mimic the way neurons function in the human brain. And this neural type, she says, is just “blowing other MT out of the water.”
So what makes it so cool? Well, remember how MT needed a separate engine to translate into each language direction? Neural engines don’t.
Microsoft was the first company to release a neural MT system. Google now has its own version. It “[allows] for a single system to translate between multiple languages,” Google explains on a company blog.

Suppose you want to know how to say something in Japanese, Korean and English. Before neural systems were available, computers would have required three different engines to make this happen. One would translate Japanese into English. Another would translate Korean into English. A third would convert Japanese into Korean.
And each engine would have had to start from scratch.
Now, instead of having lots of “brains” that only think in one direction, neural MT uses one big engine to store grammar, vocabulary — all of the information about translation going into and out of that language. This allows programmers to do the conversion of Japanese into Korean by using information from the first two translations (the Japanese and Korean into English).
Neural MT does not speed the translation of a brand new language. Nor does it help MT tackle more than one subject (such as food, sports, business or science) at a time. Translation companies still need you and your friends to figure those things out. That’s why Brandon says: “Remind science students that language is important. That it’s not just for the liberal artsy types. And that studying languages alongside their core science topics could lead to a rockin’ career!”
In other words, the translation industry — and a growing community of baby MT engines — need you.