Statistics: Make conclusions cautiously
Scientists disagree about how best to analyze data and how to conclude what a study’s results might mean
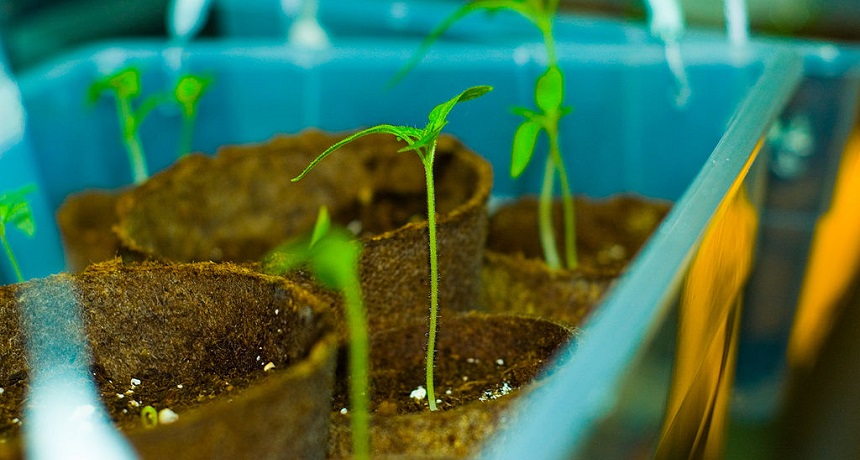
Just one experiment isn’t enough to show that one fertilizer makes a plant grow taller than another. Even with good statistics, scientists need to be careful about how they interpret their data.
Golden Hound/Wikimedia Commons
An experiment usually begins with a hypothesis — a proposed outcome or explanation for an observation. To test whether the hypothesis was right, researchers usually will conduct a series of tests, collecting data along the way. But in science, making sense of those data can be challenging. The reason: It’s a numbers game. And not all scientists will read the same meaning out of the same group of numbers.
To find out why, read on.
Let’s consider a case where scientists want to probe the effects of fertilizers. They might hypothesize that fertilizer A will produce taller plants than fertilizer B. After applying the different fertilizers to various groups of plants, the data may show that on average, the plants treated with fertilizer A indeed were taller. But this does not necessarily mean that fertilizer A was responsible for the height difference.
In science, making — and believing — such conclusions will depend on how the data stand up to a type of math known as statistics. And they start right with the original hypothesis.
Scientists will expect one treatment — here, a fertilizer — to perform differently than another. But to enter the testing without bias, scientists also need to concede that their proposed explanation might be wrong. So each hypothesis should therefore also have a corresponding null hypothesis — an understanding that there may be no change. In this experiment, a null hypothesis would hold out the prospect that the plants might respond identically to both fertilizers.
Only now are the scientists ready to run tests looking for fertilizer effects.
But for the findings of these tests to be reliable, the experiment needs to test the effects on enough plants. How many? It’s not something that scientists can guess at. So before starting the tests, the researchers must calculate the minimum number of plants they must test. And to do that, they must anticipate the chance that they could make either of two main types of errors when testing their null hypothesis.
The first, called a Type I error, is a so-called false positive. An example might be where someone concluded a fertilizer caused a difference in plant height when that treatment in fact had nothing to do with the plants’ height. A Type II error would conclude the opposite. This so-called false negative would conclude a fertilizer had no effect on plant height when in fact it did.
Scientists in many fields, such as biology and chemistry, generally believe that a false-positive error is the worst type to make. But because no experiment ever works perfectly, scientists tend to accept there is some chance an error actually will occur. If the test data indicated the chance this had happened was no higher than 5 percent (written as 0.05), most scientists in areas such as biology and chemistry would accept the findings from the experiment as being reliable.
Biologists and chemists generally consider a false negative error — here, declaring the fertilizer had no effect on plant height when it did — to be less concerning. So over time, researchers in many fields have reached a consensus that it’s fine to rely on data where there appears to be no more than a 20 percent chance that the findings represent a false-negative. This should give scientists an 80 percent chance (written 0.8) of finding a difference due to the fertilizer — if, of course, one really exists.
With these two numbers, 5 percent and 80 percent, scientists will calculate how many plants they will need to treat with each fertilizer. A mathematical test called a power analysis will supply the minimum number of plants they will need.
Now that a scientist knows the minimum number of plants to test, he or she is now ready to put some seeds in the soil and start applying the fertilizer. They may measure each plant at regular intervals, chart the data and carefully weigh all the fertilizer to be used. When the tests are over, the researcher will compare the heights of all plants in one treatment group against those in the other. They might then conclude that one fertilizer makes plants grow taller than another fertilizer.
But that may not be true. For why, read on.
More statistics, please . . .
When comparing plant heights in the two treatment groups, scientists will be looking for a discernable difference. But if they detect a difference, they’ll need to probe the likelihood that it’s real — meaning that it was likely due to something other than chance. To check that out, they need to do some more math.
Actually, the scientists will be hunting for what they call a statistically significant difference in the groups. Since the starting hypothesis had been that the fertilizers would affect the heights of treated plants, that’s the feature those scientists will examine. And there are several mathematical tests that can be used to compare two or more groups of plants (or cookies or marbles or any other things) that a scientist might wish to measure. The goal of these math tests is to judge how likely it is that any difference would be the result of chance.
One such math test is an analysis of variance. It compares how much groups of measurements overlap when there are more than two groups being measured.
Such mathematical tests yield a p value. That is the likelihood that any observed difference between groups is as large, or larger, than the one that might have been due solely to chance (and not from the fertilizer being tested). So, for example, if scientists see a p value of 0.01 — or 1 percent — that means they would expect to see a difference at least this big only 1 percent of the time (once in every 100 times they performed this experiment).
Scientists generally will rely on data where the p value is less than 0.05, or 5 percent. In fact, most scientists well consider a result that shows a p value or less 5 percent to be statistically significant. For the fertilizer example, that would suggest there would be a 5 percent chance or less of seeing the recorded difference if the fertilizers had no effect on plant heights.
This p value of 0.05 or less is the value widely sought in test data by laboratories, at science fairs and in the scientific findings reported in papers for a broad range of fields, from anesthesia to zoology.
Still, some scientists challenge the usefulness of relying on this number.
Among those critics are David Colquhoun of University Collect London and David Cox of the University of Oxford, in England. Both have pointed out that when scientists find a difference with a p value of less than 0.05, there is not just a 5 percent chance that a Type I error has occurred. In fact, they point out, there is also up to a 20 percent chance a Type II error also might have occurred. And the effect of these errors can add up as the tests are repeated over and over.
Each time, the p value for the data will be different. In the end, for any one experiment yielding a p value of less than 0.05, all that researchers can say is that they have a reason to suspect the apparent difference in treatment groups is due to the fertilizers. But scientists can never say with certainty that the fertilizer caused the difference. They can say only that in this test, there was a 5 percent chance of witnessing a difference as big or bigger in plant height if fertilizer had no effect.
And there’s more . . .
Scientists also can misinterpret the risk that a Type I — or false-positive — error has occurred. They may see a p value of 0.05 as suggesting that there is no more than a 5 percent chance they will have turned up a difference “due to the fertilizer” when none exists.
But this is not true. The researchers may simply lack enough evidence to figure out whether there is no difference due to the fertilizer.
It’s easy to think there that two negatives — no evidence and no difference — would make a positive. But no evidence of no difference is not the same as evidence for a difference.
There also can be a problem with how scientists interpret the p value. Many scientists celebrate when the analysis of their results reveals a p value of less than 0.05. They conclude there is a less than 5 percent chance that any differences in plant height are due to factors other than the one being tested. They believe that a p value of less than 0.05 means their experiment confirmed their hypothesis.
In fact, that is not what it means.
A statistically significant difference does not indicate that the test detected a true effect. It merely quantifies the chance of seeing a difference as big or bigger than the observed one (if there actually was no difference due to what was being tested).
Finally, the presence of a difference — even a statistically significant one — does not mean that difference was important.
For instance, one fertilizer may indeed result in taller plants. But the change in plant height could be so small as to have no value. Or the plants may not be as productive (for instance, yield as many flowers or fruit) or be as healthy. A significant difference does not by itself show that some measured difference is important for function.
Former Science News editor-in-chief and blogger Tom Siegfried has written two great blog posts about problems with the way many scientists do statistics. There also are articles at the end of this post that can give you more information.
Follow Eureka! Lab on Twitter
Power Words
control A part of an experiment where there is no change from normal conditions. The control is essential to scientific experiments. It shows that any new effect is probably due to only the part of the test that a researcher has altered. For example, if scientists were testing different types of fertilizer in a garden, they would want one section of to remain unfertilized, as the control. Its area would show how plants in this garden grow under normal conditions. And that give scientists something against which they can compare their experimental data.
hypothesis A proposed explanation for a phenomenon. In science, a hypothesis is an idea that must be rigorously tested before it is accepted or rejected.
null hypothesis In research and statistics, this is a statement assuming that there is no difference or relationship between two or more things being tested. Conducting an experiment is often an effort to reject the null hypothesis, or to suggest that there is a difference between two or more conditions.
p value (in research and statistics) This is the probability of seeing a difference as big or bigger than the one observed if there is no effect of the variable being tested. Scientists generally conclude that a p value of less than five percent (written 0.05) is statistically significant, or unlikely to occur due to some factor other than the one tested.
statistics The practice or science of collecting and analyzing numerical data in large quantities and interpreting their meaning. Much of this work involves reducing errors that might be attributable to random variation. A professional who works in this field is called a statistician.
statistical analysis A mathematical process that allows scientists to draw conclusions from a set of data.
statistical significance In research, a result is significant (from a statistical point of view) if the likelihood that an observed difference between two or more conditions would not be due to chance. Obtaining a result that is statistically significant means there is a very high likelihood that any difference that is measured was not the result of random accidents.
Type I error In statistics, a Type I error is rejecting the null hypothesis, or concluding that a difference exists between two or more conditions being tested, when in fact there is no difference.
Type II error (in statistics) A finding thatthat there is no difference between two or more conditions being tested, when in fact there is a difference. It’s also known as a false negative.
variable (in mathematics) A letter used in a mathematical expression that may take on more than one different value. (in experiments) A factor that can be changed, especially one allowed to change in a scientific experiment. For instance, when measuring how much insecticide it might take to kill a fly, researchers might change the dose or the age at which the insect is exposed. Both the dose and age would be variables in this experiment.