AI learned how to influence humans by watching a video game
After learning to play Overcooked, artificial intelligence could work with human teammates
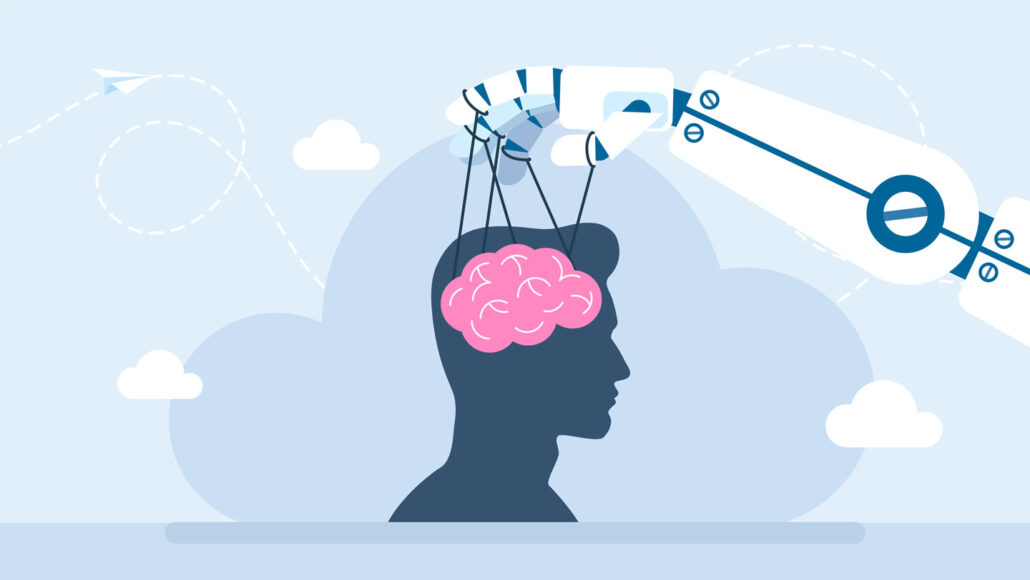
A new study shows how AI can learn to influence how people behave.
Yurii Karvatskyi/Stock / Getty Images Plus
This is another in a year-long series of stories identifying how the burgeoning use of artificial intelligence is impacting our lives — and ways we can work to make those impacts as beneficial as possible.
If you’ve ever cooked with someone, you know it requires coordination. One person chops this. The other mixes that. Sometimes, you might hand each other ingredients or tools when you’d like something done. But how might a robot handle this type of teamwork? A recent study offers some clues.
Artificial intelligence, or AI, watched people play the game Overcooked. By doing this, the AI model was able to master the game. But then it went further. It had also learned how it could nudge its human teammates to make better decisions.
Researchers shared those findings last December. They presented the work at the Neural Information Processing Systems meeting. It was held in New Orleans, La.
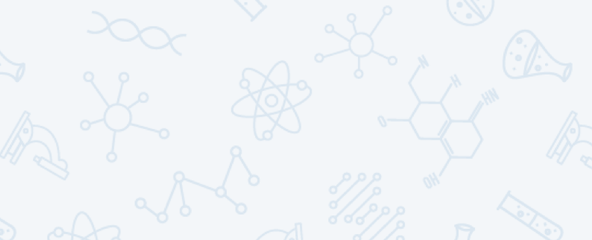
Educators and Parents, Sign Up for The Cheat Sheet
Weekly updates to help you use Science News Explores in the learning environment
Thank you for signing up!
There was a problem signing you up.
This study tackles “a crucial and pertinent problem,” says Stefanos Nikolaidis. That is: How can AI learn to influence people. Nikolaidis wasn’t involved in the new work. But he does study interactive robots at the University of Southern California in Los Angeles.
In the future, people will likely work more and more closely with AI. Sometimes, we may want AI to help guide our choices, like any good teammate would. But we also want to be able to tell when AI is affecting our choices in ways we don’t like. Some people might try to design AI to act this way. Or, someday, AI might decide to do this on its own.
For those reasons, it’s key to find out how — and how much — AI can learn to sway how people make decisions.
Learn by watching
For the new study, a group at the University of California, Berkeley, taught AI to play Overcooked. In this video game, two players work together to make and serve meals. To train AI players, the researchers first gathered data from pairs of people playing the game. Then they used those data to teach AI to play in four different ways.
In the first training method, the AI just mimicked what it saw human players do. In the second, the AI mimicked moves made by the best human players. The third training method ignored the human data. Here, two AIs learned by practicing with each other. The fourth method used a technique called offline reinforcement learning, or RL.
In offline RL, AI learned to play Overcooked by watching human teams. But it didn’t just mimic their moves. It pieced together the best bits of what it saw. That allowed it to actually play better than the humans it had watched.
After training, AIs played Overcooked with people. Those teams played two versions of the game. One was the “human-deliver” version. In it, a team earned double points if the human partner served the food. The other version was the “tomato-bonus” version. Here, teams earned double points if they served soup with tomato and no onion.
Crucially, the humans were never told the double-point rules. So the AIs had to nudge their human teammates to follow these hidden rules.
In the human-deliver game, teams with AI that had been trained using offline RL scored an average 220 points. That was about 50 percent more points than the next best AI-training methods. In the tomato-bonus game, teams with AI trained by offline RL scored an average 165 points — or about double what other teams scored.
A closer look at the AI trained with offline RL revealed one reason its teams did so well. When the AI wanted its human partner to deliver the food, it would place a dish on the counter near the human.
This may sound simple. But the AI did not see any examples of people doing this during its training. It had seen someone put down a dish. And there were times it saw someone picking up a dish. But it seems the AI figured out the value of stitching these acts together: Doing so got its human partner to serve the food and earn double points.
Nudging human behavior
Strategic dish placement may have allowed an AI to influence a human’s single next move. But could AI figure out — then influence — a human’s overall strategy, involving more than just one follow-up step?
To find out, the Berkeley team tweaked the AI model. It now based its choices on more than just the current state of the game. The AI was instructed to also now consider its partner’s past actions to work out their upcoming game plan.
Both the original and tweaked AI models learned Overcooked using offline RL. The two models then played Overcooked with human partners. The one that could figure out its partner’s strategy scored roughly 50 percent more points. In the tomato-bonus game, for instance, the AI learned to keep blocking the onions until its partner left them alone.
Do you have a science question? We can help!
Submit your question here, and we might answer it an upcoming issue of Science News Explores
Rohan Paleja is impressed that AI could learn to play Overcooked by watching past human games. Other types of reinforcement learning involve AI working with human tutors. But for many tasks, that can be time-consuming or even risk harm to those human trainers. AI developers can also build computer models that act like human tutors to teach AI. But building models that realistically mimic what people do is quite difficult.
The method here — where AI learns by watching — is “applicable to a lot of real-world problems,” Paleja says. This computer scientist works at the Lincoln Laboratory in Lexington, Mass. It’s part of the Massachusetts Institute of Technology.
For better or worse
In the future, we may be working with AI partners in kitchens, warehouses and hospitals. AI that can coax people into making better choices “could be helpful in supporting people to reach their goals when they don’t know the best way to do this,” says Emma Brunskill. She’s a computer scientist at Stanford University in California. She did not take part in the new study.
AI could look at data from health apps to nudge people toward meeting their goals, Brunskill suggests. Or it might help coax people to donate more to charity.
But such influence can have a darker side, too. “Online recommender systems can, for example, try to have us buy more,” Brunskill says. “Or watch more TV.”
Even if AI means to help, it may manipulate us in ways we don’t like, adds Micah Carroll. He’s a computer scientist at UC Berkeley, but he did not take part in the new study.
Carroll points to how AI kept blocking a human’s path in the Overcooked game. This behavior may be fine in a video game where the AI is just trying to help its team score points. But imagine an AI that blocks people’s access to other places or information. That could be dangerous, even if the AI thinks it’s doing what is best for people.
The new study shows it’s already possible to build AI that thinks it knows best and can guide people to follow its lead. Scientists don’t yet have good ways for people to tell AI what types of influence they are okay with, Carroll says. “For example, ‘I’m okay with an AI trying to argue for a specific strategy — but not forcing me to do it if I don’t want to.’”