Soccer-playing robots show how nimble AI-powered machines can be
Controlled by reinforcement learning — a type of AI — they can navigate tough terrain
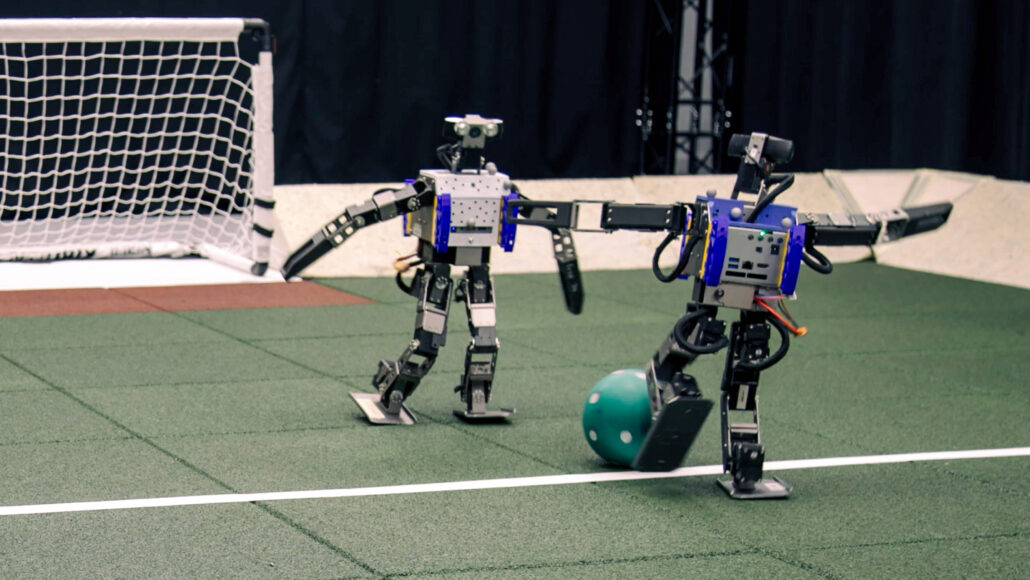
Two small, humanoid robots play soccer after being trained with reinforcement learning. This type of AI helps the robots be more agile and resilient, compared with those trained using traditional computer code.
ChatGPT and other artificial-intelligence tools are already reshaping our digital lives. But our AI interactions are now about to get physical.
Humanoid robots powered by a certain type of AI could better sense and react to the world than past machines. Such agile robots might lend a hand in factories, space stations and beyond.
The type of AI in question is called reinforcement learning. And two recent papers in Science Robotics highlight its promise for giving robots humanlike agility.
“We’ve seen really wonderful progress in AI in the digital world with tools like GPT,” says Ilija Radosavovic. This computer scientist works at the University of California, Berkeley. “But,” he adds, “I think that AI in the physical world has the potential to be even more.”
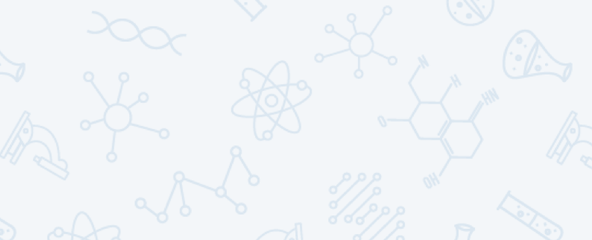
Educators and Parents, Sign Up for The Cheat Sheet
Weekly updates to help you use Science News Explores in the learning environment
Thank you for signing up!
There was a problem signing you up.
Until now, software for controlling two-legged robots has often used something called model-based predictive control. Here, the robot’s “brain” relies heavily on preset instructions for how to move around. The Atlas robot made by the company Boston Dynamics is one example of this. It’s graceful enough to do parkour. But robots with brains like this require a lot of human skill to program. And they don’t adapt well to novel conditions.
Reinforcement learning may be a better approach. In this setup, an AI model doesn’t follow preset instructions. It learns how to act on its own, through trial and error.
“We wanted to see how far we can push reinforcement learning in real robots,” says computer scientist Tuomas Haarnoja. He works at Google DeepMind in London, England. In one of the new studies, he and his team developed an AI system to control a 20-inch-tall toy robot called OP3. They wanted to teach it not only to walk, but also to play one-on-one soccer.
Soccer bots
“Soccer is a nice environment to study general reinforcement learning,” says team member Guy Lever. It requires planning, agility, exploration and competition. He, too, works at Google DeepMind in London.
The toy size of the robots they trained allowed the team to run lots of experiments on their machines and learn from their mistakes. (Larger robots are harder to operate and repair.) But before using their AI software to control any real robots, the team trained it on virtual bots.
This training came in two stages. In the first, the team trained one AI model just to get a virtual robot up off the ground through reinforcement learning. This type of learning also helped a second AI model figure out how to score goals without falling over.
The two AI models could “sense” where a virtual robot would be by knowing the positions and movements of its joints. The AI models also knew the location of everything else in this virtual world.
The AIs could use those data to direct the virtual robot’s joints to new positions. If those movements helped the robot play well, then the AI models received a virtual reward. And that encouraged them to use those same behaviors more.
In the second stage, researchers trained a third AI model to mimic each of the first two. It also learned how to score against other versions of itself.
To prepare that multi-skilled AI model to control real-world robots, the researchers changed up different aspects of its virtual world.
For instance, they randomly changed how much friction the virtual robot experienced. Or added random delays to its sensory inputs. The researchers also rewarded the AI for not just scoring goals, but also for other useful actions. For instance, the AI model got a reward for not twisting the virtual robot’s knee too much. This encouraged motions that would help avoid real-world knee injuries.
Do you have a science question? We can help!
Submit your question here, and we might answer it an upcoming issue of Science News Explores
Finally, the AI was ready to handle real robots. Those controlled by this AI walked nearly twice as fast as those controlled by software trained using preset instructions. The new AI-based robots also turned three times as fast. And they took less than half as much time to stand up.
They even showed more advanced skills. For instance, these robots could smoothly string together a series of actions.
“It was really nice to see more complex motor skills,” says Radosavovic. The AI software didn’t just learn single soccer moves. It also learned the planning required to play the game — such as knowing to stand in the way of an opponent’s shot.
“The soccer paper is amazing,” says Joonho Lee. This roboticist works at ETH Zurich in Switzerland and did not take part in the study. “We’ve never seen such resilience from humanoids,” he says.
Going life-sized
In the second new study, Radosavovic and his colleagues worked with a machine. They trained an AI system to control a humanoid robot, called Digit. It stands about 1.5 meters (5 feet) tall and has knees that bend backward, like those on an ostrich.
This team’s approach was similar to Google DeepMind’s. Both groups trained their AIs using reinforcement learning. But Radosavovic’s team used a certain type of AI model called a transformer. This type is common in large language models, such as those that power ChatGPT.
Large language models take in words to learn how to write more words. In much the same way, the AI that powered Digit looked at past actions to learn how to act in the future. Specifically, it looked at what the robot had sensed and done for the previous 16 snapshots of time. (In total, that covered roughly one-third of a second.) From those data, the AI model planned the robot’s next action.
Like the soccer-playing software, this AI model first learned to control virtual robots. In the digital world, it had to traverse terrain that included not only slopes but also cables that the robot could trip over.
After training in the virtual world, the AI system took over a real robot for a full week of outdoor tests. It didn’t fall over once. Back in the lab, the robot could withstand having an inflatable exercise ball thrown at it.
In general, the AI system controlled this robot better than traditional software did. It easily walked across planks on the ground. And it managed to climb a step, even though it hadn’t seen steps during training.
A leg (or two up)
In the last few years, reinforcement learning has become popular for controlling four-legged robots. These new studies show the same techniques also works with two-legged machines. And they show that robots built on reinforcement learning perform at least as well as — if not better than — those using preset instructions, says Pulkit Agrawal. He’s a computer scientist at the Massachusetts Institute of Technology in Cambridge. He was not involved in either of the new studies.
Future AI robots may need the dexterity of Google DeepMind’s soccer players. They may also need the all-terrain skill that Digit showcased. Both are required for soccer. And that, Lever says, “has been a grand challenge for robotics and AI for quite some time.”
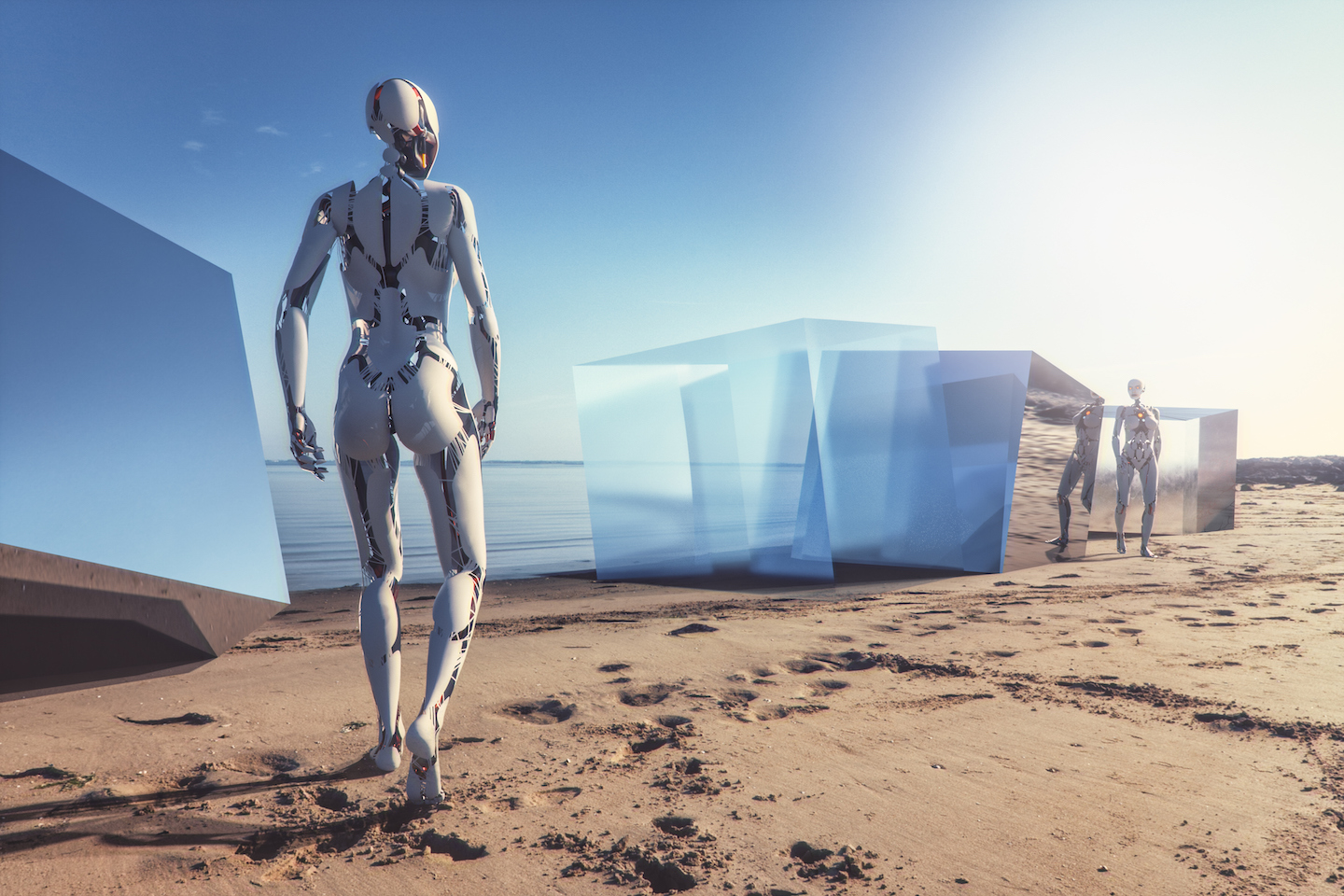