Here’s why AI like ChatGPT probably won’t reach humanlike understanding
This type of artificial intelligence isn’t good at applying what it learns to new situations
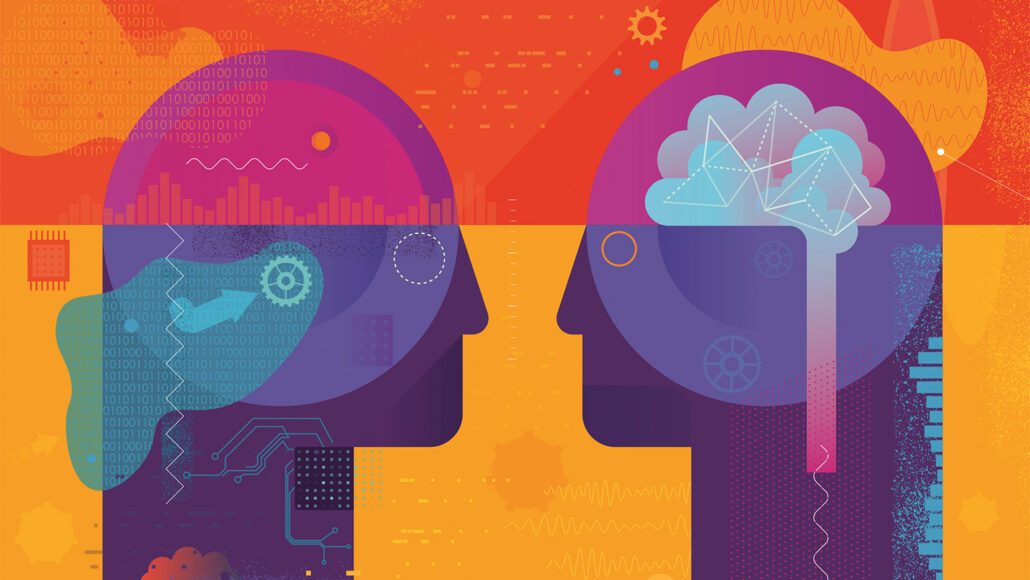
As impressive as they seem, the latest computer brains usually can’t apply all the many things that they know to situations that differ from those they encountered in training. This, however, comes easily to us.
DrAfter123/GETTY IMAGES
By Tom Siegfried and Maria Temming
If you ask ChatGPT whether it thinks like a human, this chatbot will tell you that it doesn’t. “I can process and understand language to a certain extent,” ChatGPT writes. But “my understanding is based on patterns in data, [not] humanlike comprehension.”
Still, talking to this artificial intelligence, or AI, system can sometimes feel like talking to a human. A pretty smart, talented person at that. ChatGPT can answer questions about math or history on demand — and in a lot of different languages. It can crank out stories and computer code. And other similarly “generative” AI models can produce artwork and videos from scratch.
“These things seem really smart,” said Melanie Mitchell. She’s a computer scientist at the Santa Fe Institute in New Mexico. She spoke at the annual meeting of the American Association for the Advancement of Science. It was held in Denver, Colo., in February.
AI’s increasing “smarts” have a lot of people worried. They fear generative AI could take people’s jobs — or take over the world. But Mitchell and other experts think those fears are overblown. At least, for now.
The problem, those experts argue, is just what ChatGPT says. Today’s most impressive AI still doesn’t truly understand what it is saying or doing the way a human would. And that puts some hard limits on its abilities.
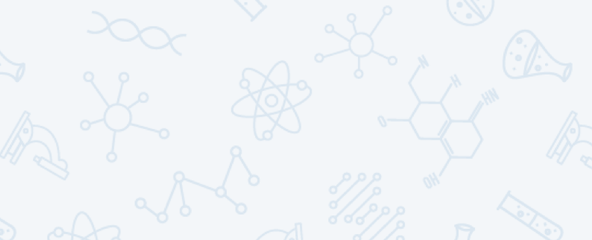
Educators and Parents, Sign Up for The Cheat Sheet
Weekly updates to help you use Science News Explores in the learning environment
Thank you for signing up!
There was a problem signing you up.
Concerns about AI are not new
People have worried for decades that machines are getting too smart. This fear dates back to at least 1997. That’s when the computer Deep Blue defeated world chess champion Garry Kasparov.
At that time, though, it was still easy to show that AI failed miserably at many things we do well. Sure, a computer could play a mean game of chess. But could it diagnose disease? Transcribe speech? Not very well. In many key areas, humans remained supreme.
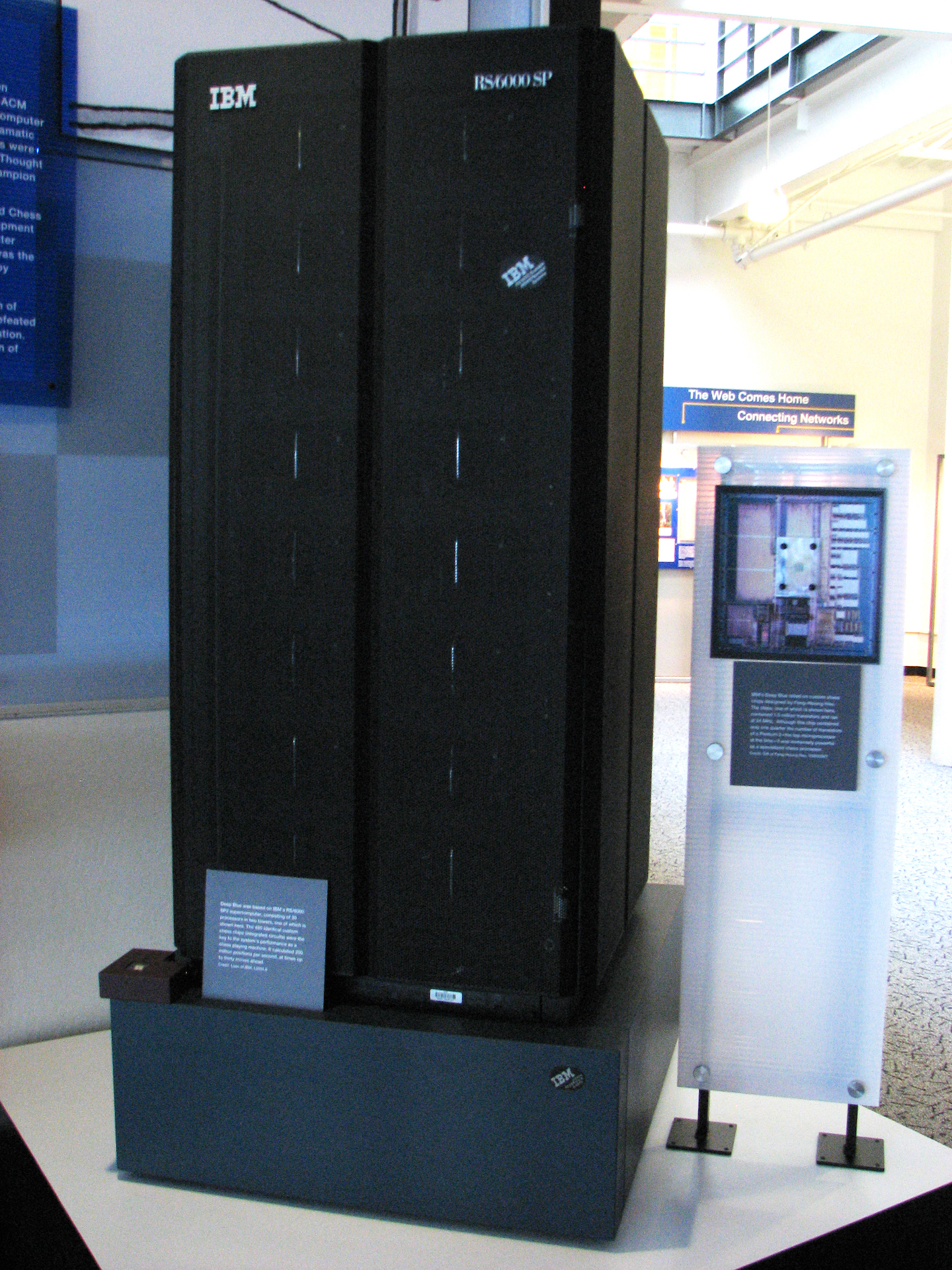
About a decade ago, that began to change.
Computer brains — known as neural networks — got a huge boost from a new technique called deep learning. This is a powerful type of machine learning. In machine learning, computers master skills through practice or looking at examples.
Suddenly, thanks to deep learning, computers rivaled humans at many tasks. Machines could identify images, read signs and enhance photographs. They could even reliably convert speech to text.
Yet those abilities had their limits. For one thing, deep-learning neural networks could be easy to trick. A few stickers placed on a stop sign, for example, made an AI think the sign said “Speed Limit 80.” Such “smart” computers also needed extensive training. To pick up each new skill, they had to view tons of examples of what to do.
So deep learning produced AI models that were excellent at very specific jobs. But those systems couldn’t adapt that expertise very well to new tasks. You couldn’t, for instance, use an English-to-Spanish AI translator for help on your French homework.
But now, things are changing again.
“We’re in a new era of AI,” Mitchell says. “We’re beyond the deep-learning revolution of the 2010s. And we’re now in the era of generative AI of the 2020s.”
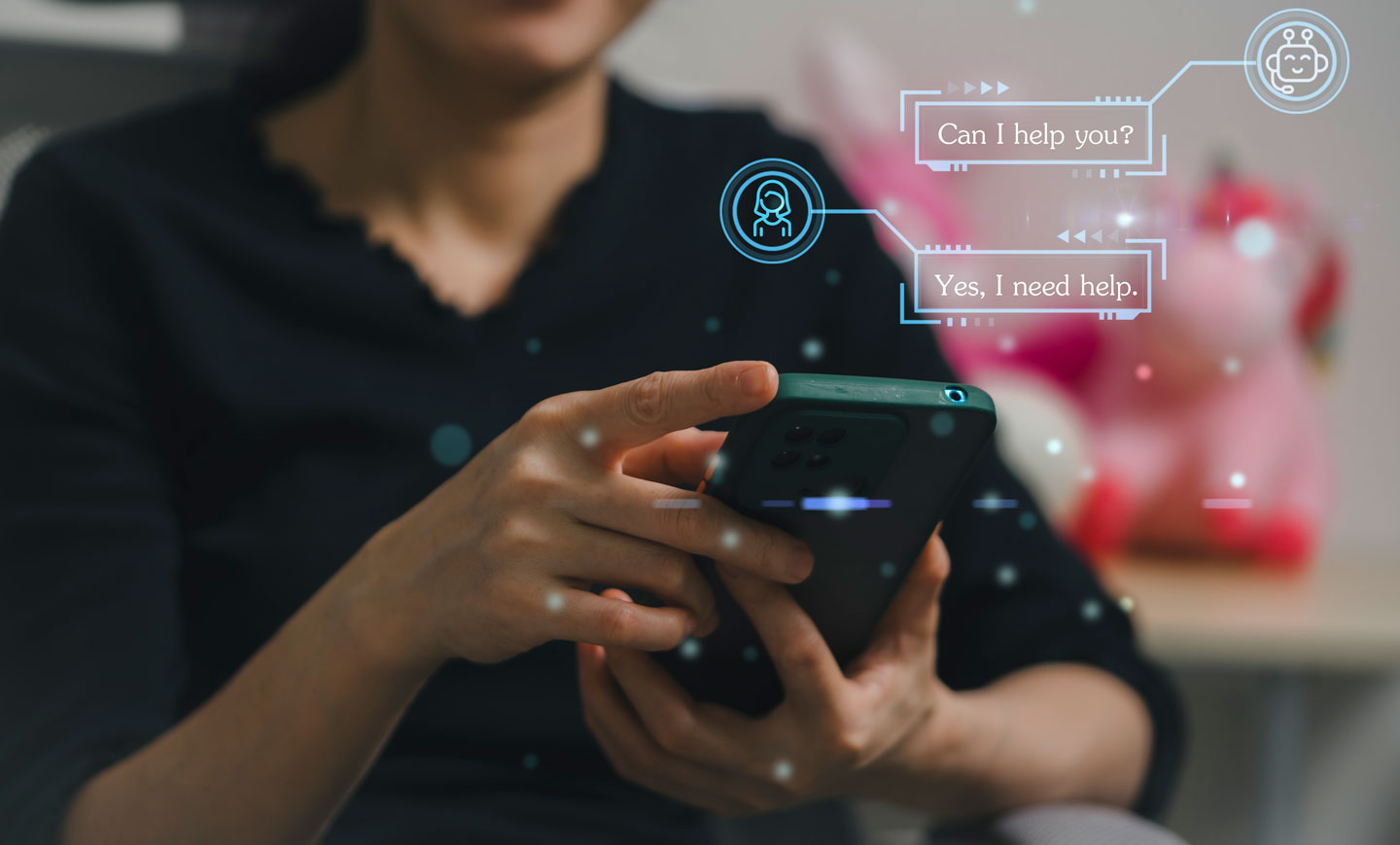
This gen-AI era
Generative AI systems are those that can produce text, images or other content on demand. This type of AI can generate many things that long seemed to require human creativity. That includes everything from brainstorming ideas to writing poems.
Many of these abilities stem from large language models — LLMs, for short. ChatGPT is one example of tech based on LLMs. Such language models are said to be “large” because they are trained on huge amounts of data. Essentially, they study everything on the internet, which includes scanned copies of countless print books.
“Large” can also refer to the number of different types of things LLMs can “learn” in their reading. These models don’t just learn words. They also pick up phrases, symbols and math equations.
By learning patterns in how the building blocks of language are combined, LLMs can predict in what order words should go. That helps the models write sentences and answer questions. Basically, an LLM calculates the odds that one word should follow another in a given context.
This has allowed LLMs to do things such as writing in the style of any author and solving riddles.
Some researchers have suggested that when LLMs accomplish these feats, they understand what they’re doing. Those researchers think LLMs can reason like people or could even become conscious in some sense.
But Mitchell and others insist that LLMs do not (yet) really understand the world. At least, not as humans do.
Narrow-minded AI
Mitchell and her colleague Martha Lewis recently exposed one major limit of LLMs. Lewis studies language and concepts at the University of Bristol in England. The pair shared their work at arXiv.org. (Studies posted there have usually not yet been vetted by other scientists.)
LLMs still do not match humans’ ability to adapt a skill to a new situation, their new paper shows. Consider this letter-string problem. You start with one string of letters: ABCD. Then, you get a second string of letters: ABCE.
Most humans can see the difference between the two strings. The final letter in the first string is replaced with the next letter of the alphabet in the second string. So when humans are shown a different string of letters, such as IJKL, they can guess what the second string should be: IJKM.
Most LLMs can solve this problem, too. That’s to be expected. The models have, after all, been well trained on the English alphabet.
Do you have a science question? We can help!
Submit your question here, and we might answer it an upcoming issue of Science News Explores
But say you pose the problem with a different alphabet. Perhaps you jumble up the letters in our alphabet to be in a different order. Or you use symbols instead of letters. Humans are still very good at solving letter-string problems. But LLMs usually fail. They are not able to take the concepts they learned with one alphabet and apply them to another. All the GPT models tested by Mitchell and Lewis struggled with these kinds of problems.
Other similar tasks also show that LLMs don’t do well in situations they weren’t trained for. For that reason, Mitchell doesn’t believe they show what humans would call “understanding” of the world.
The importance of understanding
“Being reliable and doing the right thing in a new situation is, in my mind, the core of what understanding actually means,” Mitchell said at the AAAS meeting.
Human understanding, she says, is based on “concepts.” These are mental models of things like categories, situations and events. Concepts allow people to determine cause and effect. They also help people predict the likely results of different actions. And people can do this even in situations they haven’t seen before.
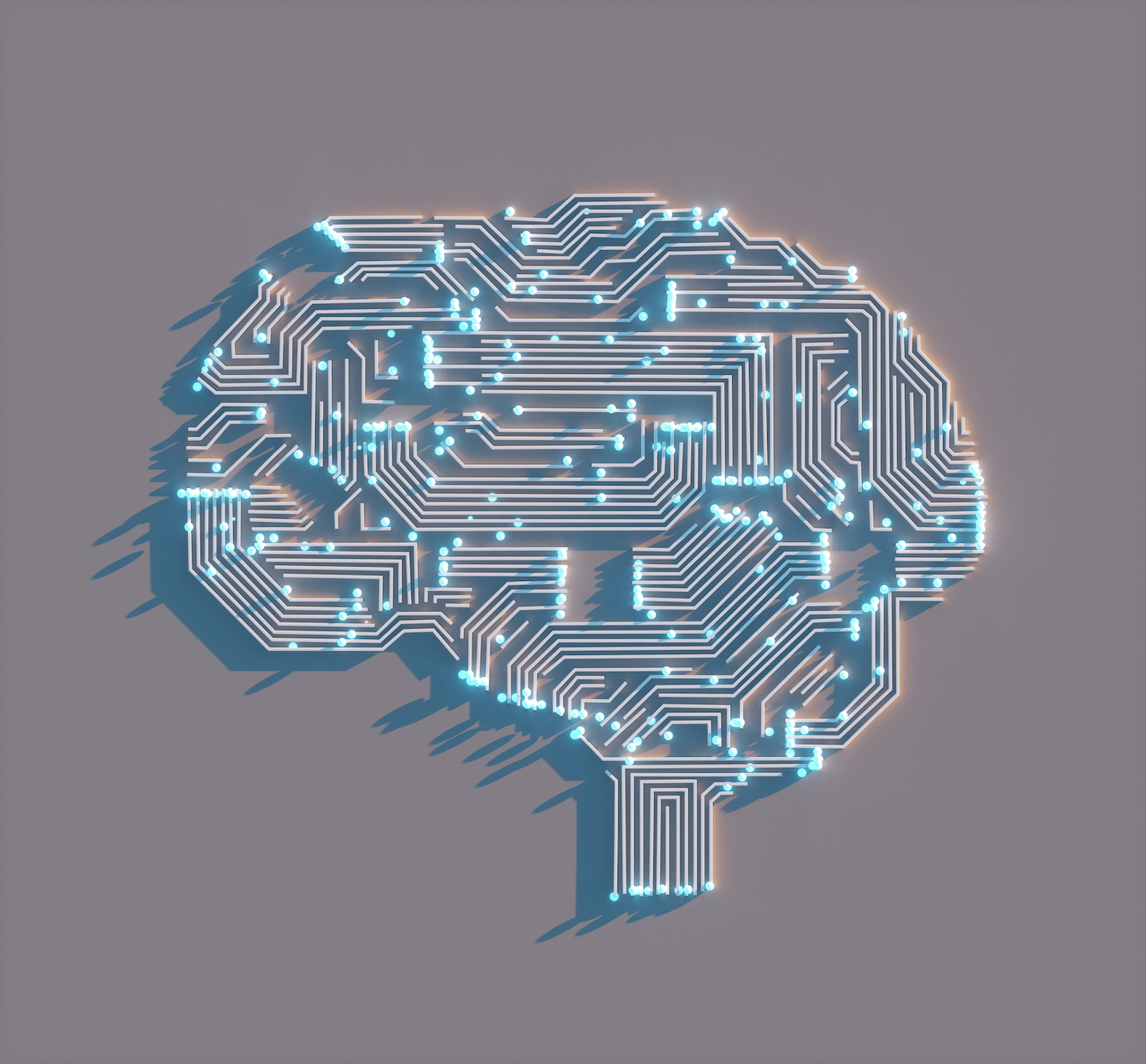
“What’s really remarkable about people … is that we can abstract our concepts to new situations,” Mitchell said.
She does not deny that AI might someday reach a level of intelligent understanding similar to humans. But machine understanding may turn out to be different from human understanding, she adds. Nobody knows what sort of technology might achieve that understanding. But if it’s anything like human understanding, it will probably not be based on LLMs.
After all, LLMs learn in a way opposite to humans. These models start out learning language. Then, they try to use that knowledge to grasp abstract concepts. Human babies, meanwhile, learn concepts first and then the language to describe them.
So talking to ChatGPT may sometimes feel like talking to a friend, teammate or tutor. But the computerized number-crunching behind it is still nothing like a human mind.