Someday soon, smartwatches may know you’re sick before you do
New wearable tech can detect signs of developing illness and put out a warning
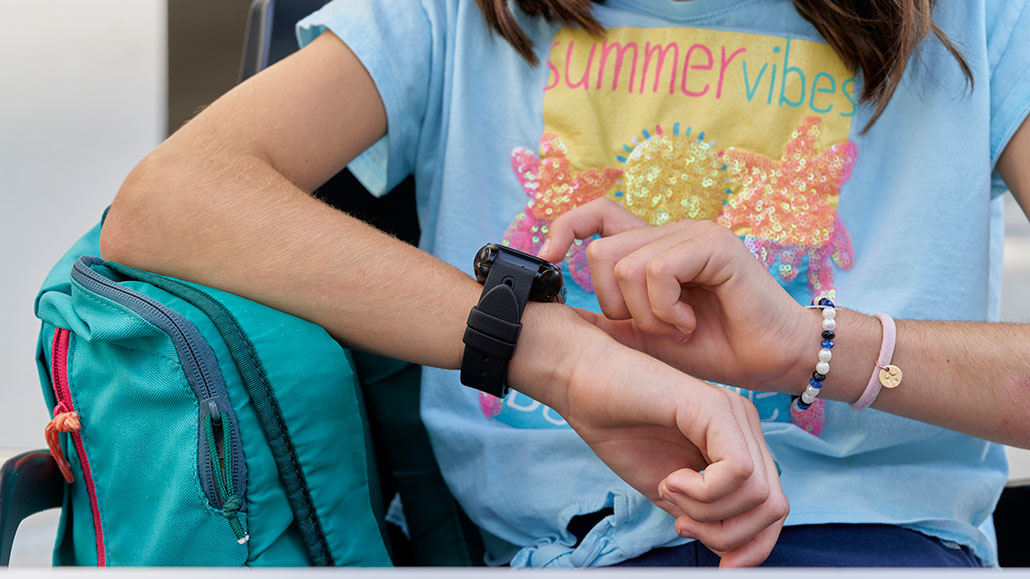
Smartwatch-style wristbands may soon be able to gauge whether you’re coming down with a cold, flu or other infection — even before you feel sick. This early warning can help you avoid spreading the disease.
aire images/Moment/Getty Images Plus
We’ve had weather forecasts for decades. Forecasting our near-term health is far tougher. Yet knowing early that we may be coming down with the flu or COVID-19 could be immensely helpful. The good news: Wearable technology, such as smartwatches, is beginning to provide just such early warnings.
Jessilyn Dunn is a biomedical engineer at Duke University in Durham, N.C. She was part of a team that analyzed heart rates and other data from wearable devices. The smartwatch-like systems contain sensors. These collect data — lots and lots of them — that can point to health or disease.
Dunn’s team asked 49 volunteers to wear sensor-laden wristbands before and after they received a cold or flu virus. At least once per second, these wristbands recorded heart rates, body movements, skin temperatures and more. In nine out of every 10 recruits, these data showed signs of developing illness at least a day before symptoms emerged.
The researchers described their findings September 29 in JAMA Network Open.
This early warning, says Dunn, can help nip infections in the bud. It may head off severe symptoms that otherwise would send vulnerable people into hospitals. And knowing you’re sick before you have symptoms can warn you to lay low so you can reduce the chance of spreading your disease.
However, these systems aren’t yet ready for the real world, notes virologist Stacey Schultz-Cherry. She works at St. Jude Children’s Research Hospital in Memphis, Tenn. “This is exciting but also very preliminary,” says Schultz-Cherry. “Much more work is needed before this approach can be rolled out on a larger scale.”
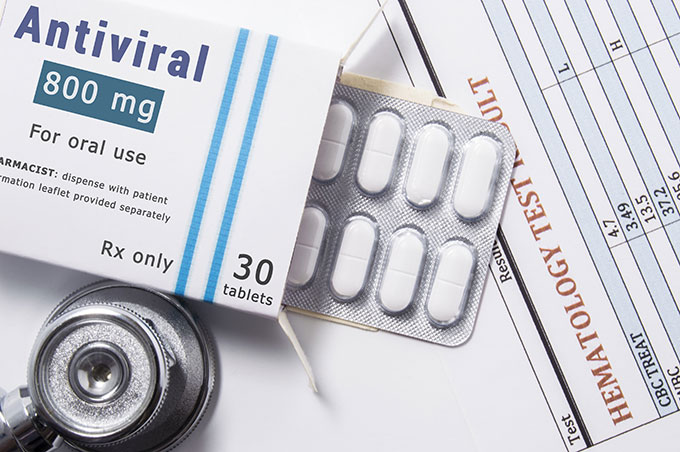
Sifting through mountains of data
The researchers gave 31 of the 49 recruits nose drops with a flu virus. The remaining people were exposed to a common cold virus.
Trials where volunteers agree to receive a virus are unusual, notes Schultz-Cherry. They also can be dangerous. So the researchers made sure the volunteers were healthy and would not give the flu to others. (Doctors also checked in on them frequently during the trial.)
Dunn’s group wanted to compare the sensor data from infected and noninfected people. But deciding who was infected “involved a substantial debate within our team,” notes Emilia Grzesiak. She’s a data scientist who worked on the project while at Duke. The team’s final decision? Recruits were infected if they reported at least five symptoms within five days of receiving the virus. A PCR test also had to detect the virus on at least two of those days.
Recruits started wearing the wristbands before they were exposed. This provided baseline data while the volunteers were healthy. The sensors continued to collect data for several days after the exposure. Some data were measured more than 30 times per second. That means the 49 recruits had up to 19 million data points each, notes Grzesiak. A computer sifted through these mountains of data in search of patterns that signaled emerging disease.
For that sifting, the computer needed an algorithm. Grzesiak developed those step-by-step instructions. Her algorithm tested all possible combinations of sensor data and time points. It looked for the biggest difference between infected and noninfected people. One example of a winning combo: Summing the average heart rate 6 to 7 hours after virus exposure and the average time between heartbeats 7 and 9 hours after exposure. (The actual best model was more complex.)
Grzesiak used some of the data to build a computer model. She tested its predictions in the remainder of the data. Then she repeated this process many times. Her final model accurately predicted infections nine times in every 10.
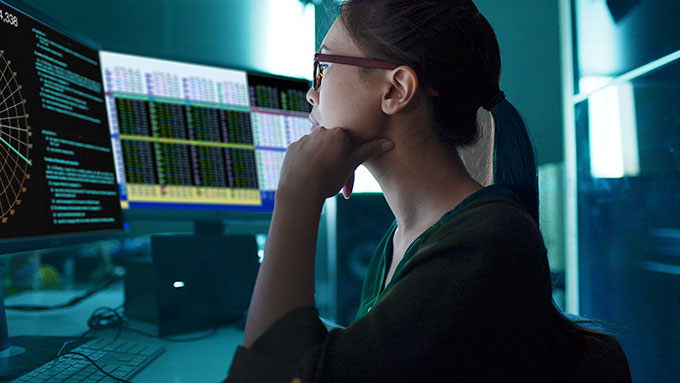
Challenges ahead
One challenge is that many viral infections have similar symptoms. In fact, many things other than viruses trigger the same symptoms. Examples, Schultz-Cherry notes, include food poisoning, asthma or seasonal allergies. Similarly, heart rates respond to things that have nothing to do with infections. Examples include exercise and scary movies.
What’s more, in real life, we don’t know who was exposed to some virus and when. So that telltale post-exposure time window won’t be known. Potentially infected people might be those whose data exceed a certain value in any two-hour window. But Dunn’s team has not yet tested how well the prediction model would work in this setting.
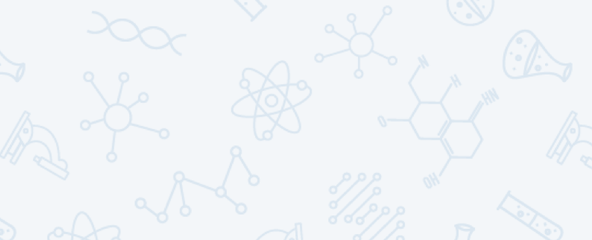
Educators and Parents, Sign Up for The Cheat Sheet
Weekly updates to help you use Science News Explores in the learning environment
Thank you for signing up!
There was a problem signing you up.
Could such a system one day point to people coming down with COVID-19? Maybe, says Benjamin Smarr. He’s a bioengineer at the University of California, San Diego. Similar technologies, he notes, are being developed elsewhere to provide early warnings of that infection.
Such studies sound exciting. But much work is left to do. For instance, Smarr notes, prediction accuracies of 95 percent sound good. But that number means “telling one out of every 20 people every night that they will get the flu when they actually won’t.”
Smarr expects continued improvements in prediction accuracies. Future models will likely include other types of bodily changes that pinpoint developing illness. And researchers will be fine-tuning those models by analyzing how well they predict effects in thousands of people.
This story is one in a series presenting news on technology and innovation, made possible with generous support from the Lemelson Foundation.